Archive for category CFEngine
Fixing 7digital’s Music Search, Part Two: The 88% Speed Improvement
Posted by Michael Okarimia in 7digital, API, Automation, CFEngine, DevOps, search, SOLR, Vagrant, Virtualization on August 18, 2014
I’m Michael Okarimia, and I’m Team Lead Developer of the Content Discovery Team at 7digital, starting from Jan 2014. The Content Discovery team recently improved the catalogue & search infrastructure for the 7digital API. This post is the second in a series which explains how we turned resolved many of the long standing problems with 7digital’s music search and catalogue platform. If you haven’t read the first post in the series, which explains the problem of making 27 million tracks searchable, go and start reading from there.
In this post I detail how we improved the track search service so it’s response times reduced by 88%.
How we fixed track search: From 2014 onwards
As discussed in the previous post in this series, the data store used for searching tracks was a Lucene index being used as a document store, totalling 660gb in size. It was clearly far too large, and a scalable alternative had to be found. We also had to solve the inconsistency problems what arose due to the search index never being as up to data as the SQL database used to power the rest of the platform. These problems manifested themselves to API users as sporadic internal server errors when ever they wanted to stream or purchase a track that was returned from a search result.
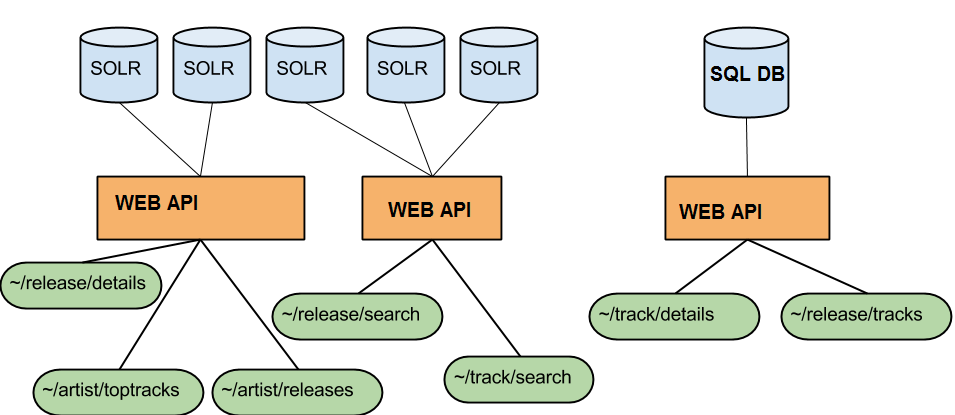
Search and Catalogue API endpoints with their different databases. This caused inconsistencies, but it increases availability
Rather than using Lucene as both a document store and a search index, we decided to create a new search index based on the searchable fields only, and use it to resolve 7digital track IDs. Armed with the track IDs that matched the search terms, we could do a look up on the catalogue database and then return that as the result. At the time we thought this should fix the inconsistency problem, and perhaps make searching faster.
Given that we were all relatively new to the team, we did some through investigation of the the search platform. We discovered that the previous schema in production was indexing fields like track price which were never actually searched upon. Additionally all of the twenty nine fields were stored, which was needed because it was used as document store. This increased the size of the index.
We created a prototype and came up with a much smaller search index, around 10 Gb in size compared with 660Gb. This new index contained only the searchable fields, such as track title, artist name and release title, reducing the schema down from twenty nine indexed fields to just nine.
When querying the new smaller index, the response would only contain the track IDs. However the response from the track search API endpoint is more than a list of track IDs. It is enriched with ample track metadata that can be used to build results in a search results page. There had to be a second step to fetch this data.
The returned IDs would then be used to look up the details of the track (such as it’s title, price and release date), and then the track details were composed into a single, ordered list of search results. The meant that the modified track/search endpoint would then return that list, which looked exactly like the old track/search responses that were returned from the giant index.
There was already an API endpoint that returned the contents of a track, namely, the ~/track/details endpoint. When given a 7digital track ID it returns all the available track meta data, such as track title, artist name, release date, etc. It’s response lacked some of the fields we require that were returned in the track search responses, so we added them so it now returns the price and available formats of a track.
We built this second index and deployed it to production in parallel with the gargantuan 660Gb one. One by one we rewrote the search & catalogue API endpoints so they instead used the smaller index to fetch matching track IDs and then called the ~/track/details endpoint to populate the results.
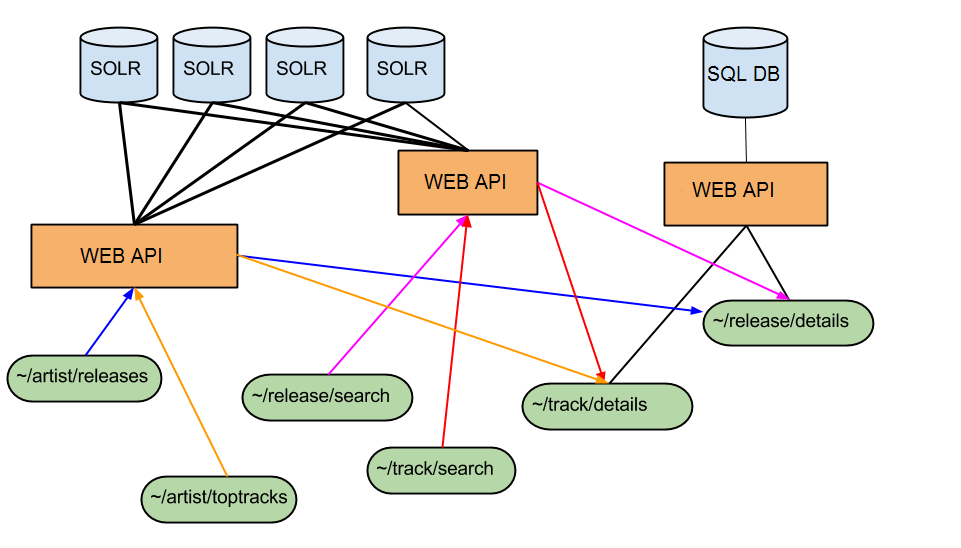
Catalogue and Search Endpoints as of July 2014. All responses are populated from the SQL DB which is most up to date. There are no longer inconsistencies
To test that the schema changes worked we learned that the best way was to use Vagrant and Test kitchen prior to pushing the schema to our continuous integration platform. We used CFEngine configuration management tool to define our SOLR schemas with the configuration file edited locally on a developer’s machine. Next we spun up a Vagrant instance that would apply the configuration defined in the file, and would then run Server Spec tests to verify some of the basic functionality of the SOLR server, using the altered schema. This arrangement was informed by our prior work to test infrastructure code, which I previously blogged about. Once the initial schema changes were known work on SOLR, we pushed the new schema up onto our continuous integration platform, something which Chris O’Dell has blogged about more eloquently than I could. Part our deployment pipeline then ran acceptance tests against the API endpoints which use this index, verifying that existing functionality was intact.
Using this technique, we migrated our APIs that relied upon this gargantuan 660Gb index to use the new smaller one instead. At the time of writing it is 100 times smaller, weighing in at 6.3 Gb.
The new smaller search index came with a number of advantages;
- being reliant on the ~/track/details endpoint meant we always returned current results, and we were 100% consistent with the rest of the API, which eliminated the catalogue inconsistencies problem;
- we could create a brand new index within an hour, meaning up to date data;
- much faster average response times for ~/track/search, reduced the response time by 88% from around 2600ms to around 350ms;
- no more deleted documents bloating the index, thus reducing the search space;
- longer document cache and filter cache durations, which would only be purge at the next full index every 12 hours, this helped performance;
- quicker to reflect catalogue updates, as the track details were served from the SQL database which could be updated very rapidly.
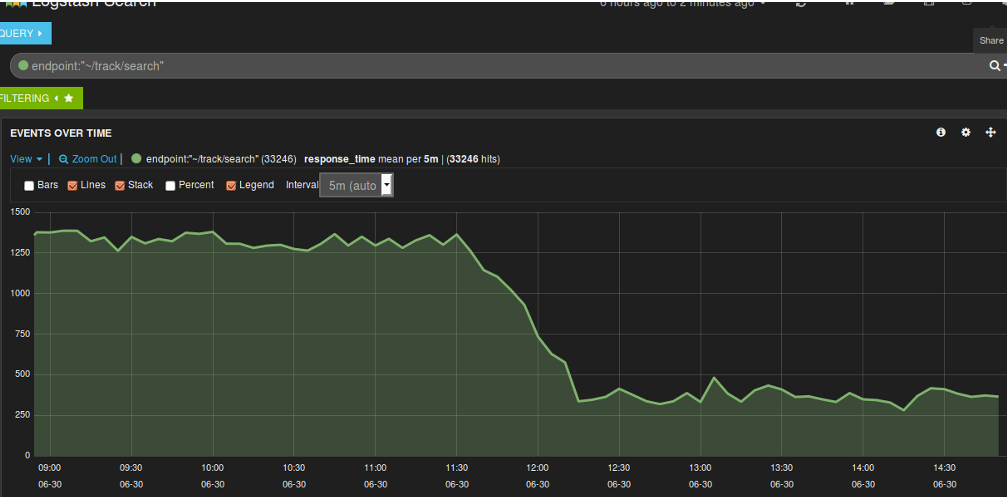
Y Axis units in milliseconds. Once we used a smaller index and enabled filter caching, the response time dropped.
Now that the entire index could fit in the memory of the server hosting it, this gave advantages such as:
- faster document retrieval;
- no more long JVM running garbage collection processes causing performance issues and server connection time outs. These were triggered replication when the index was changed, this now only occurred twice a day when the index was rebuilt;
- The platform could now cope with more traffic, based our our load tests using JMeter
Searching for Music Releases
We have also started this strategy for our release search endpoint. This endpoint performs searches for on the metadata of a music release based on the search terms. For example, one can search for either an artist or a release title. This endpoint queries a Lucene index containing data about music releases which is used as a massive document store as well as a search index. There are around 2.7 million releases in 7digital’s catalogue that are searchable.
We have integrated a call to the ~/release/details endpoint for each release ID returned in the results of a query to the ~/release/search index. This makes the endpoint always consistent with the catalogue DB, which fixed the inconsistency problem we had when searching for releases. At the time of writing we haven’t yet built a smaller release index which would improve response time, but this work is already under way.
We aim to reduce the size of the release index too and be able to build a new one multiple times a day.
For our track index, creating a new index of the music catalogue on a regular basis meant we could take advantage of the index time features that SOLR offers. I’ll write about how we used those features in a future post.
What we learnt
Never* Avoid, if possible incrementally add or delete documents to or from a Lucene index without rebuilding it regularly. Otherwise you will have an index filled with unsearchable but deleted documents which will bloat the index. This means you will inexorably experience search query times slowing over time;Never* Avoid using Lucene as a document database, it won’t scale to work effectively with millions of richly annotated documents;- Make sure your index can fit in the memory of the server it’s hosted on;
- Index as few Lucene fields as possible;
- Store as few Lucene fields as possible;
- Replication will invalidate your SOLR caches, and will trigger long running JVM garbage collections, try to minimise how often it happens;
- If you are using a Master/Slave SOLR configuration, slaves with differing replication polling intervals will lead to inconsistent responses when you eventually query the oldest non-replicated slave;
- Storing your index on Solid State Drives will speed up query time;
- Regularly rebuild your index to keep it small;
If you’re in interested in some more context of how we decided upon the implementation, my colleague Dan Watts has an excellent write up on his blog, detailing some of the problems that I mention in this post.
*Updated my opinion on how to Lucene upon reflection of Nick Tune’s comments.